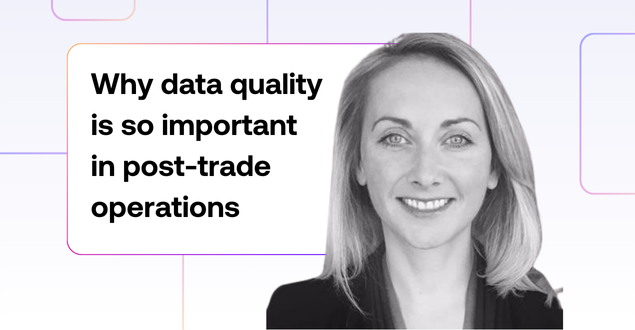
Table of Contents
The critical role of data quality in post-trade efficiency for T+1 settlement
Global financial institutions face mounting pressure to adapt with significant shifts such as compressed settlement cycles, requiring faster trade processing, tighter controls and better operational efficiency. Data quality is key for success in this environment. It’s not just about meeting regulatory requirements or avoiding fines; it’s about enabling growth, reducing risk, and positioning your organization for long-term success.
But why is data quality so important in post-trade operations? And how can institutions prepare for the challenges ahead? This blog explores the role of data quality in post-trade efficiency, the challenges the industry faces specifically with T+1, and actionable solutions to overcome them.
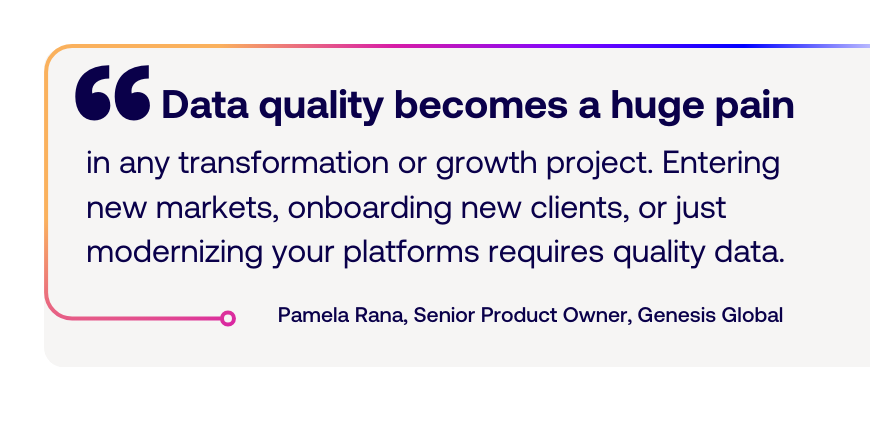
Why data quality is the foundation of T+1 success
Transitioning to a T+1 settlement drastically compresses the post-trade timeline. In a T+2 environment, trades are typically booked by around 5 p.m. on T+0 and must be confirmed by around 7 p.m. on T+1—giving operations teams roughly 26 hours to complete all post-trade processes, including allocations, communications and tax agreements. Under T+1, however, all of these processes must now be completed by 7 p.m. on T+0, with regulations imposing fines if not done by 9 p.m. This leaves as little as a two-hour window. That means one thing for trade operations teams across the globe: there’s zero room for error.
Quality data ensures trades are matched, allocated and confirmed on time, reducing risks like failed settlements and regulatory penalties. Poor data, on the other hand, can derail operations. According to BaseCap Analytics, bad data can cost businesses millions.
Furthermore, with a surge in market data volumes over the last few years, this issue is only getting larger. The Trade highlights that global markets saw data volumes rocket from 100 petabytes daily in 2020 to over 300 petabytes by 2024. Therefore, managing, integrating and ensuring the quality of this data is key to market survival.
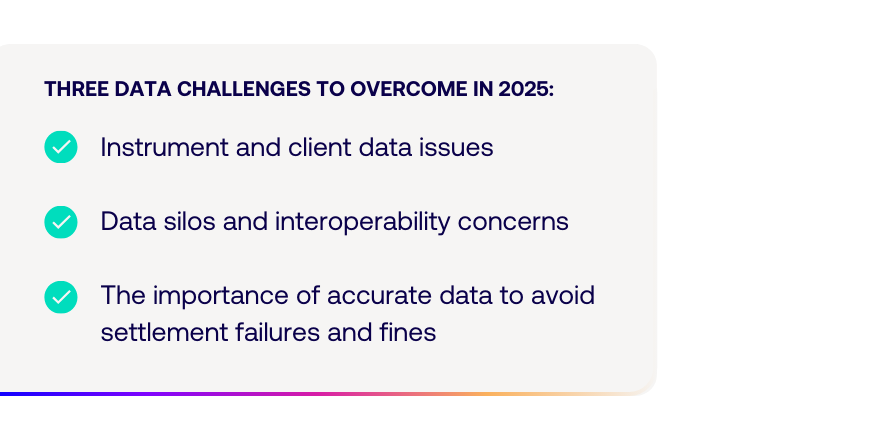
Key data quality challenges in T+1 settlement
1. Data silos and lack of system interoperability
Data fragmentation across siloed systems complicates trade processing. Middle-office operations often rely on legacy systems that don’t integrate seamlessly with one another. This creates bottlenecks for trade matching, especially when multiple platforms come into play.
Plus, manual interventions caused by siloed data can introduce errors and enhance risk, especially under a compressed timeline.
2. Client data inconsistencies
Accurate client data is essential for seamless trade matching and settlement. Inconsistencies in client information, such as mismatched account numbers or outdated Standing Settlement Instructions (SSIs), can lead to settlement failures and increased operational risk.
SSIs, which dictate how trades are settled, often suffer from issues like being stored in multiple formats or managed by various firms, leading to manual interventions and delays. To mitigate these challenges, firms should aim to centralize client data management, ensuring that all systems access a single, up-to-date source of truth. Implementing automated processes for updating and disseminating client information can significantly reduce errors and enhance efficiency in the settlement process
3. Settlement failures and regulatory risks
Meeting T+1 commitments requires efficient, error-free post-trade workflows. Yet, settlement failure rates remain a concern. Reports from BNP Paribas show that data inconsistencies were a driving factor in non-CNS fails, which stayed elevated at 2.92% after T+1 implementation, compared to 2.01% under T+2. Such failures trigger fines and damage relationships with both clients and regulators.
The problem is particularly acute when trade instructions or client preferences are missing or incorrect. It leads to manual corrections being rushed through. By the settlement deadline, even small errors can snowball into larger consequences.
Solutions to enhance data quality for T+1
To address these challenges head-on, financial institutions must adopt modern tools and strategies that ensure high data accuracy, system agility and streamlined workflows.
Simplify your processes
Simplification and real-time integration are an essential part of enhancing your data quality. Eliminating manual, fragmented processes in favor of streamlined and simplified workflows reduces errors and accelerates post-trade operations. Implementing real-time APIs not only facilitates data flow between front, middle, and back-office systems but also enables access to a well-maintained golden source of reference data. This significantly enhances data quality by ensuring consistency across systems and reduces the time required to process new accounts that involve updates in multiple platforms. As a result, the reliance on manual interventions is minimized, improving overall efficiency and reducing settlement delays.
Prioritize automation and straight-through processing (STP)
Automation is no longer optional under T+1. From trade matching to exception handling, automated systems ensure timelines are met. Experts from Societe Generale caution that manual errors in compressed timelines exacerbate risks. Solutions with built-in automation can reduce reliance on manual workflows, freeing middle-office teams to focus on strategic initiatives. For example, TAM centralizes trade data and automates post-trade workflows, significantly reducing delays in matching and confirmations.
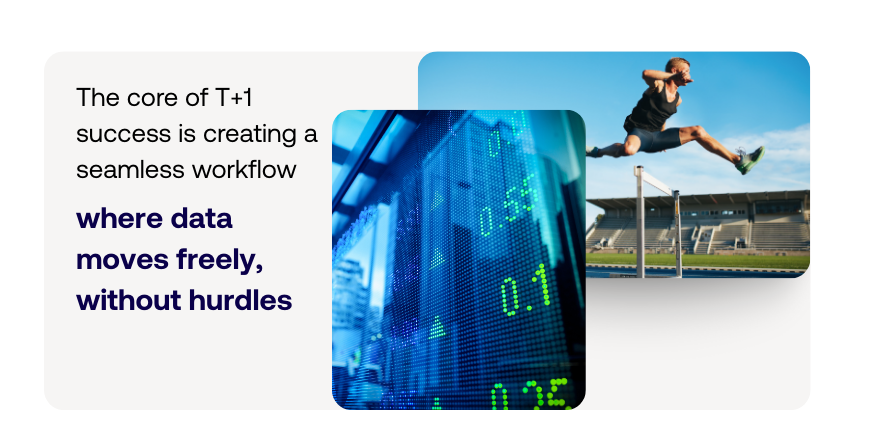
Maintain a golden source of data
In an era where real-time information is the norm, maintaining separate data silos across front, middle, and back offices is no longer sustainable. Firms must invest in interoperable systems that offer real-time data sharing and visibility. The key to operational efficiency lies in having a single, high-quality source of truth that feeds all systems instantly and consistently.
“The core of T+1 success lies not just in standalone fixes, but in creating a seamless workflow where data moves freely, without hurdles.” – Pamela Rana
It’s 2025, why are we still duplicating data across platforms instead of keeping them in sync? A unified, golden source not only aligns teams but produces a more resilient trade lifecycle.
Standardize standing settlement instructions (SSIs)
Consistent SSI data reduces mismatches and simplifies settlement workflows. By combining automation with standardization, institutions improve matching rates and minimize manual interventions. This is a vital step toward achieving true compliance and avoiding costly fines.
Let data enhance, not restrict, your workflows
One standout post-trade solution is a platform that removes unnecessary data restrictions. Tools like Genesis’s Trade Allocation Manager (TAM) are designed to integrate seamlessly into existing workflows by offering flexibility around certain aspects of the data flow. This allows middle-office teams to streamline processes without being bound by the rigid data requirements often seen in front-office platforms. For instance, TAM enables firms to migrate and scale data operations while maintaining their front-office systems, supporting a smoother path to T+1 readiness without requiring simultaneous overhauls.
Pamela Rana emphasizes this approach:
“With TAM, you can scaffold your systems, migrate the middle office, and improve processes without touching the front office. It’s a low-risk, high-reward solution.”
Why data quality matters more than ever
“If you don’t get the data right, you’re not settling on time. The buy-side doesn’t care if it’s data quality or system issues; the middle office is still on the hook.” – Pamela Rana, post-trade expert
Data quality is a firm-wide priority that prevents missed settlements, client dissatisfaction, and significant financial losses. When firms prioritize data quality, they not only avoid fines and compliance issues but also create opportunities for growth. Expanding into new markets, onboarding new clients, and adopting innovative matching services all rely on clean, accurate data.
But how can firms efficiently take action? By adopting modern solutions like Genesis’s Trade Allocation Manager (TAM). TAM enhances trade settlement efficiency, enables workflow scalability, and reduces operational risks by centralizing post-trade processes and automating data management. TAM accelerates allocations and confirmations, ensuring that trade data is consistent and error-free.
If your organization struggles with fragmented workflows or outdated systems, now is the time to modernize. Learn more about Trade Allocation Manager (TAM) and get in touch to transform your post-trade processing today.