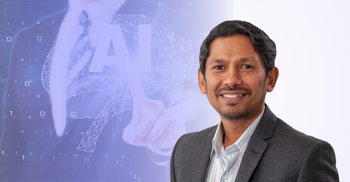
Table of Contents
- The challenge of primary market bond data management
- How AI transforms unstructured deal data processing
- Why private LLMs are ideal for institutional capital market
- Optimizing primary market operations with AI
Subscribe today to stay informed and get regular updates from Genesis Global
The challenge of primary market bond data management
For asset management firms investing in the primary market for corporate bonds, achieving a complete, real-time view of new deals coming to market and changes to deal terms is key to efficient credit analysis and rapidly placing orders for allocations.
However, syndicating banks use multiple and competing channels to disseminate data to asset managers, making it challenging to aggregate and reconcile key information. While deal platforms like DirectBooks and Ipreo (S&P Global) are major data sources, emails and instant messages still play a major role in communicating deal data to asset managers. With each deal receiving up to 30 updates across the duration of the syndication process, it’s difficult for trading desks to stay on top of the latest updates and keep portfolio managers informed.
How AI transforms unstructured deal data processing
Unlike data delivered by platforms, email and instant messages are unstructured – a syndicate bank can use a limitless variety of data formats, terms, data labels or naming conventions. There is much variability in how data is presented in email and instant messages. This is because the messages are generated by individuals, rather than systems on the bank side, using their preferred formats and terminology for variables like tenor, callability, coupon and currency. Historically, it has been difficult, if not impossible, to auto-process this data, partly because traditional parsing techniques cannot cope with the variability in deal messages.
As a result, asset managers have had to use time-consuming, error-prone manual workarounds to manage off-platform information until now. AI enables the creation of more powerful, adaptable data processors and is uniquely suited to interpreting and extracting deal information from the unstructured data contained within emails and instant messages.
Why private LLMs are ideal for institutional capital market
Most AI relies on a Large Language Model (LLM) to understand and extract meaning from text. Training an LLM for a specific task is complex and can produce varying results from the same inputs. However, careful training and fine-tuning for specific use cases can yield highly accurate results, thus making a trained LLM suitable for interpreting primary market bond data.
Despite rapid innovation in public LLMs, we believe a private LLM is best suited for most use cases in institutional capital markets. With a private model, it is easier to safeguard data, directly train the model on a specific task, calibrate its performance and control costs.
Utilizing AI for email and chat message processing provides a potent tool for obtaining a comprehensive, real-time overview of the diverse new deal market across various platforms, including on and off-platform deals. Aggregating deal data enables the creation of an integrated, deal-focused workspace that optimizes how asset managers operate in primary markets for corporate bonds.
Optimizing primary market operations with AI
In addition, the ability to process unstructured deal data can provide a more real-time view of a deal and the primary market. For example, a syndicate bank might transmit a change in coupon or other key term via email or chat before updating the deal on a platform. In that scenario, the AI-driven system could update an asset manager’s deal screen before the update is published on the platform. Similarly, AI can process grey market data to gather additional intelligence about the demand for specific new deals. When pricing windows are open for only a few hours, any advantage in making decisions and placing orders for allocations faster should benefit the asset manager.
At Genesis, we are using AI to revolutionize how asset managers conquer the corporate bond primary market by efficiently processing complex data from unstructured sources like emails and chat messages. A private LLM, custom-built for institutional capital markets, not only ensures data security and task specificity but also optimizes operations, enabling accurate and real-time decision-making in time-sensitive markets.
Start building finance-grade
applications 10x faster
Explore the Genesis Application Platform in action with a 60-day free trial* to get your first application built and in-market faster than ever before.
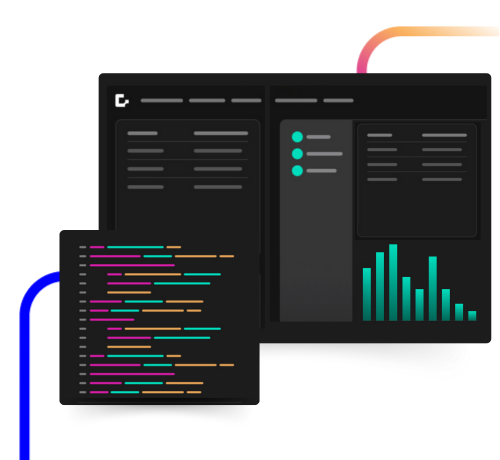